-
Golden, G., Popescu, C., Israel, S., Perlman, K., Armstrong, C., Fratila, R., Tanguay-Sela, M., Benrimoh, D. Applying artificial intelligence to clinical decision support in mental health: What have we learned? Health Policy and Technology, Volume 13, Issue 2, 2024, 100844, ISSN 2211-8837, https://doi.org/10.1016/j.hlpt.2024.100844.
-
Benrimoh D, Israel S, Fratila R, Armstrong C, Perlman K, Rosenfeld A, Kapelner A. Editorial: ML and AI Safety, Effectiveness and Explainability in Healthcare. . Frontiers Big Data. 2021 Jul 12;4:727856. doi: 10.3389/fdata.2021.727856. PMID: 34322667; PMCID: PMC8312342.
-
Benrimoh, D., Israel, S., Perlman, K., Fratila, R., & Krause, M. (2018). Meticulous Transparency —An Evaluation Process for an Agile AI Regulatory Scheme . In M. Mouhoub, S. Sadaoui, O. Ait Mohamed, & M. Ali (Eds.), Recent Trends and Future Technology in Applied Intelligence (pp. 869 –880). Springer International Publishing.
-
A new role for the private sector in responsible innovation. David E. Winickoff , Sebastian M. Pfotenhauer , Nina Frahm, David Benrimoh, Hermann Garden, Judy Iles, Thomas R. Insel, Gary E. Marchant (In press, Nature Biotechnology)
Bringing science and tech together
Aifred’s focus is using real machine learning, on real data, in a novel combination.
High quality clinical datasets are used as data input: responses to surveys from patients participating in clinical trials testing different medications. Our solution does not need expensive or time-consuming tests, therefore we can easily introduce the tool to clinics and scale rapidly. This helps us improve care quality while reducing costs. A global problem needs a global solution, building localized versions of our AI model will enable us to meet this global need.
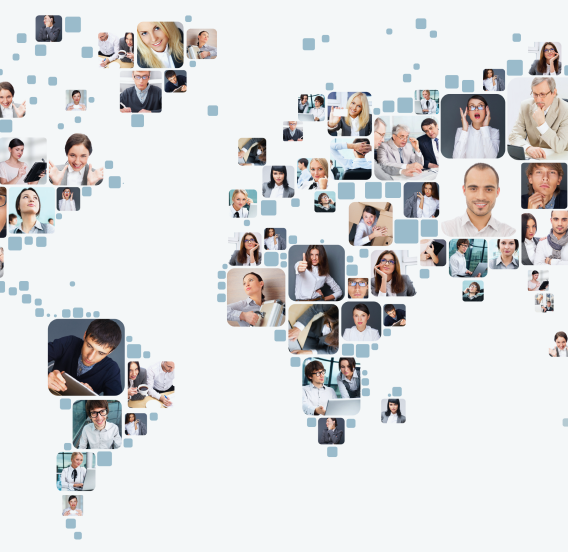
Our published research
We have created collaborative research partnerships in Canada, UK, US and Israel to further our research and are committed to the principles open innovation in the areas of basic science and the rigor of peer review processes.
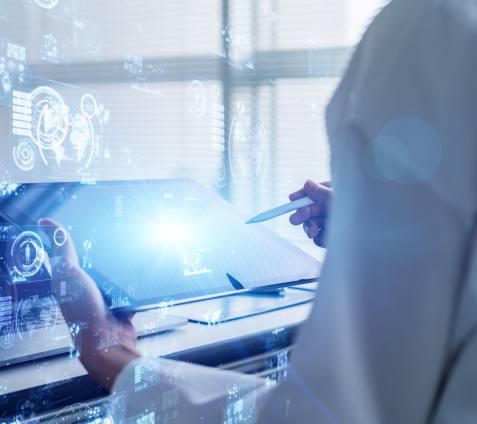
Our technology and approach is also disease-area agnostic. It can be adapted and applied beyond depression in the future, to address many global diseases where treatment selection is a challenge.
We rigorously test our models to ensure we don’t propagate biases, and we are implementing and pioneering new approaches to model interpretability, both of which are part of our commitment to AI ethics.