-
Golden, G., Popescu, C., Israel, S., Perlman, K., Armstrong, C., Fratila, R., Tanguay-Sela, M., Benrimoh, D. Applying artificial intelligence to clinical decision support in mental health: What have we learned? Health Policy and Technology, Volume 13, Issue 2, 2024, 100844, ISSN 2211-8837, https://doi.org/10.1016/j.hlpt.2024.100844.
-
Benrimoh D, Israel S, Fratila R, Armstrong C, Perlman K, Rosenfeld A, Kapelner A. Editorial: ML and AI Safety, Effectiveness and Explainability in Healthcare. . Front Big Data. 2021 Jul 12;4:727856. doi: 10.3389/fdata.2021.727856. PMID: 34322667; PMCID: PMC8312342.
-
Benrimoh, D., Israel, S., Perlman, K., Fratila, R., & Krause, M. (2018). Meticulous Transparency —An Evaluation Process for an Agile AI Regulatory Scheme . In M. Mouhoub, S. Sadaoui, O. Ait Mohamed, & M. Ali (Eds.), Recent Trends and Future Technology in Applied Intelligence (pp. 869 –880). Springer International Publishing.
-
A new role for the private sector in responsible innovation. David E. Winickoff , Sebastian M. Pfotenhauer , Nina Frahm, David Benrimoh, Hermann Garden, Judy Iles, Thomas R. Insel, Gary E. Marchant (In press, Nature Biotechnology)
Combiner sciences et technologies
L’objectif d’Aifred est de combiner l’apprentissage machine et les données du monde réel selon une approche novatrice.
Des ensembles de données cliniques de haute qualité sont utilisés - issus de réponses à des questionnaires soumis aux patients qui participent à des essais cliniques concernant différents traitements. Notre solution ne nécessite aucun test long ni coûteux, ce qui nous permet de mettre en place l’outil dans les cliniques et de l’adapter rapidement ; et ainsi d’améliorer la qualité des soins tout en réduisant les coûts. À problème global, solution globale, et c’est en concevant des versions adaptées de notre modèle d’IA que nous parviendrons à répondre à ce besoin global. AI model will enable us to meet this global need.
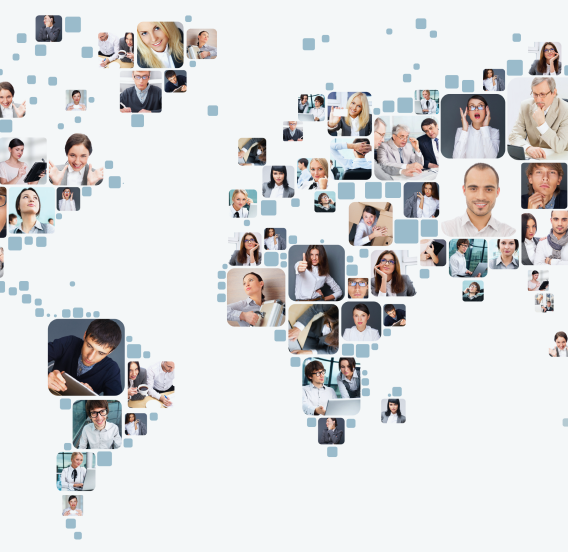
Nos recherches publiées
Nous avons établi différents partenariats de collaboration en recherche (États-Unis, Israël, Canada et Royaume-Uni) pour faire progresser nos travaux et sommes attachés aux principes d’innovation libre dans les domaines de la science fondamentale et de rigueur à travers les processus d’examen par les pairs.
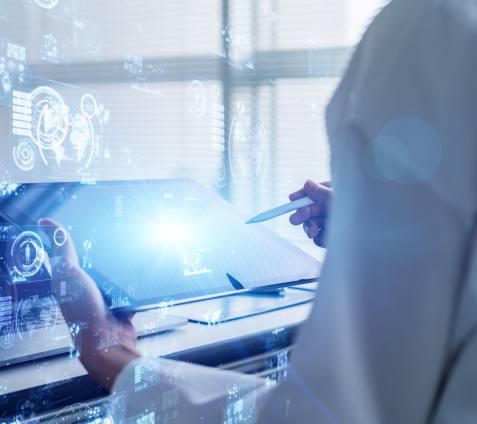
Notre technologie repose également sur une approche globale. Outre la dépression, elle pourra être adaptée et appliquée à de nombreuses autres maladies dans le monde, pour lesquelles le choix des traitements représente un défi.
Nous testons rigoureusement nos modèles, afin d’éviter la diffusion d’évaluations biaisées, et expérimentons de nouvelles approches d’interprétation - deux démarches qui sont au cœur de notre engagement en faveur de l’éthique de l’intelligence artificielle.